Note
Go to the end to download the full example code or to run this example in your browser via JupyterLite or Binder
EEG Topographic Map¶
import numpy as np
import matplotlib.pyplot as plt
#from spkit import ICA
import spkit as sp
print('spkit version :', sp.__version__)
spkit version : 0.0.9.7
Load and filter EEG Signal¶
# Load sample EEG Data ( 16 sec, 128 smapling rate, 14 channel)
# Filter signal (with IIR highpass 0.5Hz)
X, fs, ch_names = sp.data.eeg_sample_14ch()
Xf = sp.filter_X(X.copy(),fs=128.0, band=[0.5], btype='highpass',ftype='SOS')
XR = sp.eeg.ATAR(Xf.copy(),wv='db3', winsize=128, thr_method='ipr',beta=0.2, k1=10, k2=100,OptMode='elim')
Rhythmic Powers,¶
# Total
Pm = sp.eeg.rhythmic_powers(X=XR.copy(),fs=fs,fBands=[[8,14], [15,32]],Sum=True,Mean=False,SD =True)[0]
Pm = 20*np.log10(Pm)
# in windows
Pmt = sp.eeg.rhythmic_powers_win(X=XR.copy(),winsize=128*2,overlap=64,fs=fs,
fBands=[[8,14], [15,32]],Sum=True,Mean=False,SD =True)[0]
Pmt = 20*np.log10(Pmt)
Topomap of Total power¶
fig,ax = plt.subplots(1,2,figsize=(10,4))
Z1,im1 = sp.eeg.topomap(data=Pm[0],ch_names=ch_names,shownames=False,axes=ax[0],return_im=True)
Z2,im2 =sp.eeg.topomap(data=Pm[1],ch_names=ch_names,shownames=False,axes=ax[1],return_im=True)
ax[0].set_title(r'$\alpha$ : [8-14] Hz')
ax[1].set_title(r'$\beta$ : [15-32] Hz')
plt.colorbar(im1, ax=ax[0],label='dB')
plt.colorbar(im2, ax=ax[1],label='dB')
plt.suptitle('Spkit-style')
plt.show()
fig,ax = plt.subplots(1,2,figsize=(10,4))
Z1,im1 = sp.eeg.topomap(data=Pm[0],ch_names=ch_names,shownames=False,axes=ax[0],return_im=True,style='eeglab-mne')
Z2,im2 =sp.eeg.topomap(data=Pm[1],ch_names=ch_names,shownames=False,axes=ax[1],return_im=True,style='eeglab-mne')
ax[0].set_title(r'$\alpha$ : [8-14] Hz')
ax[1].set_title(r'$\beta$ : [15-32] Hz')
plt.colorbar(im1, ax=ax[0],label='dB')
plt.colorbar(im2, ax=ax[1],label='dB')
plt.suptitle('eeglab/mne-style')
plt.show()
Topomap for specific time-windows¶
t1,t2 = 7,18
plt.figure(figsize=(10,4))
plt.subplot(211)
plt.plot(Pmt[:,0,:])
plt.xlim([0,Pmt.shape[0]-1])
plt.vlines([t1,t2],ymin=Pmt[:,0,:].min()-5,ymax=Pmt[:,0,:].max(),color='k')
plt.grid()
plt.ylabel(r'$\alpha$ (dB)')
plt.subplot(212)
plt.plot(Pmt[:,1,:])
plt.xlim([0,Pmt.shape[0]-1])
plt.vlines([t1,t2],ymin=Pmt[:,0,:].min()-5,ymax=Pmt[:,0,:].max(),color='k')
plt.grid()
plt.ylabel(r'$\beta$ (dB)')
plt.xlabel('window #')
plt.text(t1,0,'t1',fontsize=15,ha='center')
plt.text(t2,0,'t2',fontsize=15,ha='center')
plt.subplots_adjust(hspace=0)
plt.show()
fig,ax = plt.subplots(1,2,figsize=(10,4))
Z1,im1 = sp.eeg.topomap(data=Pmt[t1,0,:],ch_names=ch_names,vmin=Pmt[t1].min(),vmax=Pmt[t1].max(),
shownames=False,axes=ax[0],return_im=True)
Z2,im2 =sp.eeg.topomap(data=Pmt[t1,1,:],ch_names=ch_names,vmin=Pmt[t1].min(),vmax=Pmt[t1].max(),
shownames=False,axes=ax[1],return_im=True)
ax[0].set_title(r'$\alpha$ : [8-14] Hz')
ax[1].set_title(r'$\beta$ : [15-32] Hz')
#plt.colorbar(im1, ax=ax[0],label='dB')
plt.colorbar(im2, ax=ax[1],label='dB')
plt.suptitle('t1')
plt.show()
fig,ax = plt.subplots(1,2,figsize=(10,4))
Z1,im1 = sp.eeg.topomap(data=Pmt[t2,0,:],ch_names=ch_names,vmin=Pmt[t2].min(),vmax=Pmt[t2].max(),
shownames=False,axes=ax[0],return_im=True)
Z2,im2 =sp.eeg.topomap(data=Pmt[t2,1,:],ch_names=ch_names,vmin=Pmt[t2].min(),vmax=Pmt[t2].max(),
shownames=False,axes=ax[1],return_im=True)
ax[0].set_title(r'$\alpha$ : [8-14] Hz')
ax[1].set_title(r'$\beta$ : [15-32] Hz')
#plt.colorbar(im1, ax=ax[0],label='dB')
plt.colorbar(im2, ax=ax[1],label='dB')
plt.suptitle('t2')
plt.show()
Total running time of the script: (0 minutes 0.829 seconds)
Related examples
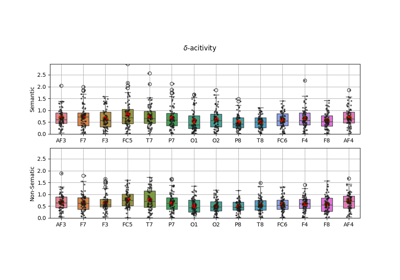
EEG Computing Rhythmic Features - PhyAAt - Semanticity
EEG Computing Rhythmic Features - PhyAAt - Semanticity