Information Theory for Real-Valued signals¶
Updating the documentation …
Entropy of signal with finit set of values is easy to compute, since frequency for each value can be computed, however, for real-valued signal it is a little different, because of infinite set of amplitude values. For which spkit comes handy.
*Following Entropy functions compute entropy based the on the sample distribuation, which by default consider process to be IID (Independent Identical Disstribuation) - which means no temporal dependency.*
*For temporal dependency (non-IID) signals, Spectral, Sample, Aproximate, SVD and Dispersion Entropy functions can be used. Which are discribed below*
Entropy of real-valued signal (~ IID)¶
Let’s first start with two signals, (two time series), x with uniform distribution, i.e., x ~ U(), and y with normal distribution, i.e., y ~ N()
import numpy as np
import matplotlib.pyplot as plt
import spkit as sp
x = np.random.rand(10000)
y = np.random.randn(10000)
plt.figure(figsize=(12,5))
plt.subplot(121)
sp.HistPlot(x,show=False)
plt.subplot(122)
sp.HistPlot(y,show=False)
plt.show()
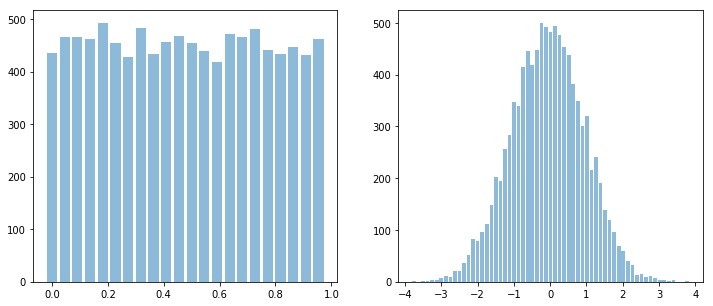
Shannan entropy¶
Shannon entropy can be computed as follow;
#Shannan entropy
H_x = sp.entropy(x)
H_y = sp.entropy(y)
print('Shannan entropy')
print('Entropy of x: H(x) = ',H_x)
print('Entropy of y: H(y) = ',H_y)
Shannan entropy
Entropy of x: H(x) = 4.4581180171280685
Entropy of y: H(y) = 5.04102391756942
Rényi entropy¶
Rényi entropy, also known as Collision Entropy. It is generalized form entropy, where shannon entropy is special case with alpha=1.
#Rényi entropy
Hr_x= sp.entropy(x,alpha=2)
Hr_y= sp.entropy(y,alpha=2)
print('Rényi entropy')
print('Entropy of x: H(x) = ',Hr_x)
print('Entropy of y: H(y) = ',Hr_y)
Rényi entropy
Entropy of x: H(x) = 4.456806796146617
Entropy of y: H(y) = 4.828391418226062
Mutual Information¶
I_xy = sp.mutual_Info(x,y)
print('Mutual Information I(x,y) = ',I_xy)
Joint Entropy H(x,y) = 9.439792556949234
Joint Entropy¶
H_xy= sp.entropy_joint(x,y)
print('Joint Entropy H(x,y) = ',H_xy)
Mutual Information I(x,y) = 0.05934937774825322
Conditional entropy¶
H_x1y= sp.entropy_cond(x,y)
H_y1x= sp.entropy_cond(y,x)
print('Conditional Entropy of : H(x|y) = ',H_x1y)
print('Conditional Entropy of : H(y|x) = ',H_y1x)
- output:
Conditional Entropy of : H(x|y) = 4.398768639379814 Conditional Entropy of : H(y|x) = 4.9816745398211655
Cross entropy¶
H_xy_cross= sp.entropy_cross(x,y)
print('Cross Entropy of : H(x,y) = :',H_xy_cross)
Cross Entropy of : H(x,y) = : 11.591688735915701
Kullback–Leibler divergence¶
D_xy= sp.entropy_kld(x,y)
print('Kullback–Leibler divergence : Dkl(x,y) = :',D_xy)
Kullback–Leibler divergence : Dkl(x,y) = : 4.203058010473213
Entropy of real-valued signal (~ non-IID)¶
For following section, we will consider three different signals to compare the Entropy,
x1 ~ \(cos(2\pi 10t)+cos(2\pi 20t)+cos(2\pi 30t)\) - sinasodal signal with three frequency componets
x2 ~ \(\mathcal{N}(0,1)\) - random gaussian signal with zero mean and sd=1
x3 ~ \(\mathcal{U}(-0.5, 0.5)\) - random signal with uniform distribution ranges from -0.5 to 0.5, zero mean
Spectral Entropy¶
Though spectral entropy compute the entropy of frequency components considering that frequency distribution is ~ IID, however, each frequency component has a temporal characteristics, so this is an indirect way to considering the temporal dependency of a signal.
fs = 1000
t = np.arange(1000)/fs
x1 = np.cos(2*np.pi*10*t)+np.cos(2*np.pi*30*t)+np.cos(2*np.pi*20*t)
np.random.seed(1)
x2 = np.random.randn(1000)
x3 = np.random.rand(1000)-0.5
Hx1_se = sp.entropy_spectral(x1,fs=1,method='welch',bining=False,normalize=False)
Hx2_se = sp.entropy_spectral(x2,fs=1,method='welch',bining=False,normalize=False)
Hx3_se = sp.entropy_spectral(x3,fs=1000,method='welch',bining=False,normalize=False)
Hx1_se_n = sp.entropy_spectral(x1,fs=1,method='welch',bining=False,normalize=True)
Hx2_se_n = sp.entropy_spectral(x2,fs=1,method='welch',bining=False,normalize=True)
Hx3_se_n = sp.entropy_spectral(x3,fs=1000,method='welch',bining=False,normalize=True)
print(' \t Spectral Entropy \t normalized ∈ [0,1]')
print('--'*30)
print(f'x1:\t {Hx1_se} \t {Hx1_se_n}')
print(f'x2:\t {Hx2_se} \t {Hx2_se_n}')
print(f'x3:\t {Hx3_se} \t {Hx3_se_n}')
output
x (signal) |
in bits |
normalized ∈ [0,1] |
---|---|---|
x1 ~ \(\mathcal{Sin}(10,20,30)\) |
2.784884691460944 |
0.39720359155165325 |
x2 ~ \(\mathcal{N}(0,1)\) |
6.887616844406343 |
0.9823696313956456 |
x3 ~ \(\mathcal{U}(-0.5,0.5)\) |
6.910233231600092 |
0.9855953700586592 |
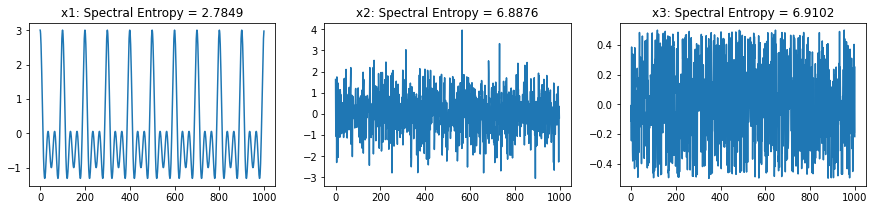
Approximate Entropy¶
Approximate Entropy is Embedding-based entropy function. Rather than considering a signal sample, it consider the m-continues samples (a m-dimensional temporal pattern) as a symbol generated from a process. This set of “m-continues samples” is considered as “Embedding” and then estimating distribution of computed symbols (embeddings). In case of a real valued signal, two embeddings will rarely be an exact match, so, the factor r is defined as if two embeddings are less than r distance away to each other, they are considered as same. This is a way to quantization of embedding and limiting the Embedding Space.
For Approximate Entropy the value of r depends the application and the order (range) of signal. One has to keep in mind that r is the distance be between two Embeddings (m-dimensional temporal pattern). A typical value of r can be estimated on based of SD of x ~ 0.2*std(x).
Hx1_apx = sp.entropy_approx(x1,m=3,r=0.2*np.std(x1))
Hx2_apx = sp.entropy_approx(x2,m=3,r=0.2*np.std(x2))
Hx3_apx = sp.entropy_approx(x3,m=3,r=0.2*np.std(x3))
print(Hx1_apx, Hx2_apx, Hx3_apx)
(0.23429427895105137, 0.5921334630488566, 0.6720444345470105)
Sample Entropy¶
Sample Entropy is a modified version of Approximate Entropy. m and r are same as in for Approximate entropy
Hx1_sae = sp.entropy_sample(x1,m=3,r=0.2*np.std(x1))
Hx2_sae = sp.entropy_sample(x2,m=3,r=0.2*np.std(x2))
Hx3_sae = sp.entropy_sample(x3,m=3,r=0.2*np.std(x3))
print(Hx1_sae, Hx2_sae, Hx3_sae)
(0.23462714901066314, 2.1931512519485836, 2.24992380933707)
Approximate Entropy Vs Sample Entropy¶
Sample entropy was proposed as the improved version of Approximate entropy, here we can compare both with a small simulation
First, lets compare the already computed values and time taken by each function
print(' \t Approx Entropy \t Sample Entropy')
print('--'*30)
print(f'x1:\t {Hx1_apx} \t {Hx1_sae}')
print(f'x2:\t {Hx2_apx} \t {Hx2_sae}')
print(f'x3:\t {Hx3_apx} \t {Hx3_sae}')
x (signal) |
Approximate Entropy |
Sample Entropy |
---|---|---|
x1 ~ \(\mathcal{Sin}(10,20,30)\) |
0.23429427895105137 |
0.23462714901066314 |
x2 ~ \(\mathcal{N}(0,1)\) |
0.5921334630488566 |
2.1931512519485836 |
x3 ~ \(\mathcal{U}(-0.5, 0.5)\) |
0.6720444345470105 |
2.24992380933707 |
Compare execution time
import time tt1 = [] for _ in range(5): start = time.process_time() sp.entropy_approx(x1,m=3,r=0.2*np.std(x1)) tt1.append(time.process_time() - start) print(time.process_time() - start) tt2 = [] for _ in range(5): start = time.process_time() sp.entropy_sample(x1,m=3,r=0.2*np.std(x1)) tt2.append(time.process_time() - start) print(time.process_time() - start) print(f'Approx Entropy:\t {np.mean(tt1)} \t {np.std(tt1)}') print(f'Sample Entropy:\t {np.mean(tt2)} \t {np.std(tt2)}')
Approximate and Sample Entropy : Time¶ Entropy fun
mean
sd
Approximate Entropy
3.11875
0.06139
Sample Entropy
0.1625
0.0159
Now, lets compute both entropy values by varies a degree of noise in a signal. This can be done by using a linear combination of x1 and x2, or x1 and x3.
ApSmEn1 = []
ApSmEn2 = []
SD = []
for i,p in enumerate(np.arange(0,1+0.04,0.05)):
sp.utils.ProgBar(i,22)
x41 = x1*(1-p) + p*x2
aprEn = sp.entropy_approx(x41,m=3,r=0.2*np.std(x41))
smEn = sp.entropy_sample(x41,m=3,r=0.2*np.std(x41))
ApSmEn1.append([p,aprEn,smEn])
x42 = x1*(1-p) + p*x3
aprEn = sp.entropy_approx(x42,m=3,r=0.2*np.std(x42))
smEn = sp.entropy_sample(x42,m=3,r=0.2*np.std(x42))
ApSmEn2.append([p,aprEn,smEn])
SD.append([p,0.2*np.std(x41),0.2*np.std(x42)])
ApSmEn1 = np.array(ApSmEn1)
ApSmEn2 = np.array(ApSmEn2)
SD = np.array(SD)
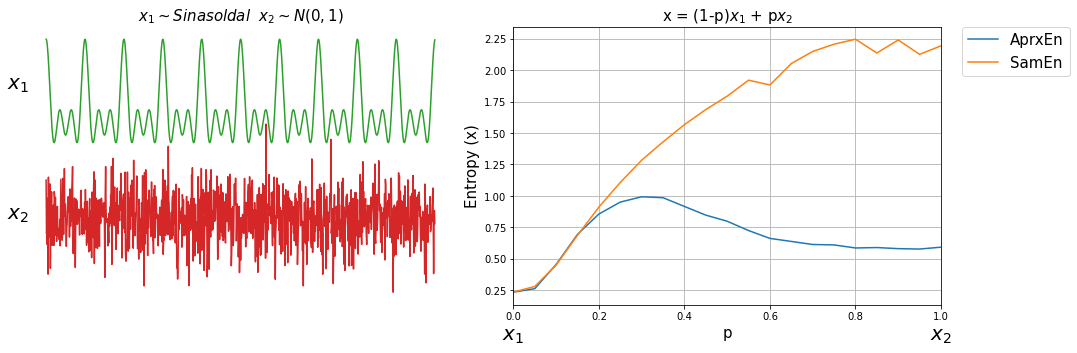
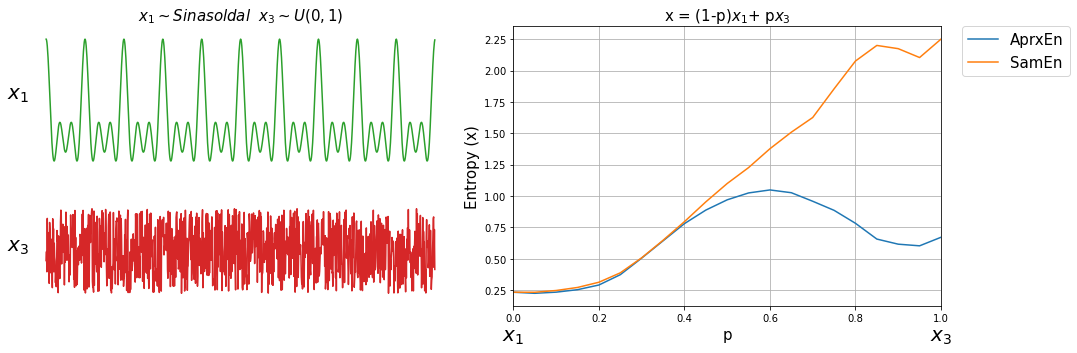
Singular Value Decomposition Entropy¶
Hx1_svd = sp.entropy_svd(x1,order=3, delay=1)
Hx2_svd = sp.entropy_svd(x2,order=3, delay=1)
Hx3_svd = sp.entropy_svd(x3,order=3, delay=1)
print(Hx1_svd, Hx2_svd, Hx3_svd)
(0.49681180617094506, 1.5847351068262792, 1.584635658386532)
Permutation Entropy¶
Hx1_prm = sp.entropy_permutation(x1,order=3, delay=1)
Hx2_prm = sp.entropy_permutation(x2,order=3, delay=1)
Hx3_prm = sp.entropy_permutation(x3,order=3, delay=1)
print(Hx1_prm, Hx2_prm, Hx3_prm)
(1.3785312756717454, 2.583366627307274, 2.5824960835257484)
x (signal) |
SVD Entropy |
Permutation Entropy |
---|---|---|
x1 ~ \(\mathcal{Sin}(10,20,30)\) |
0.49681180617094506 |
1.3785312756717454 |
x2 ~ \(\mathcal{N}(0,1)\) |
1.5847351068262792 |
2.583366627307274 |
x3 ~ \(\mathcal{U}(0,1)\) |
1.584635658386532 |
2.5824960835257484 |
Dispersion Entropy¶
For Dispersion Entropy, check the next section
Examples with EEG Signal¶
Single Channel¶
import numpy as np
import matplotlib.pyplot as plt
import spkit as sp
from spkit.data import load_data
print(sp.__version__)
# load sample of EEG segment
X,ch_names = load_data.eegSample()
t = np.arange(X.shape[0])/128
nC = len(ch_names)
x1 =X[:,0] #'AF3' - Frontal Lobe
x2 =X[:,6] #'O1' - Occipital Lobe
#Shannan entropy
H_x1= sp.entropy(x1,alpha=1)
H_x2= sp.entropy(x2,alpha=1)
#Rényi entropy
Hr_x1= sp.entropy(x1,alpha=2)
Hr_x2= sp.entropy(x2,alpha=2)
print('Shannan entropy')
print('Entropy of x1: H(x1) =\t ',H_x1)
print('Entropy of x2: H(x2) =\t ',H_x2)
print('-')
print('Rényi entropy')
print('Entropy of x1: H(x1) =\t ',Hr_x1)
print('Entropy of x2: H(x2) =\t ',Hr_x2)
print('-')
Multi-Channels (cross)¶
#Joint entropy
H_x12= sp.entropy_joint(x1,x2)
#Conditional Entropy
H_x12= sp.entropy_cond(x1,x2)
H_x21= sp.entropy_cond(x2,x1)
#Mutual Information
I_x12 = sp.mutual_Info(x1,x2)
#Cross Entropy
H_x12_cross= sp.entropy_cross(x1,x2)
#Diff Entropy
D_x12= sp.entropy_kld(x1,x2)
print('Joint Entropy H(x1,x2) =\t',H_x12)
print('Mutual Information I(x1,x2) =\t',I_x12)
print('Conditional Entropy of : H(x1|x2) =\t',H_x12)
print('Conditional Entropy of : H(x2|x1) =\t',H_x21)
print('-')
print('Cross Entropy of : H(x1,x2) =\t',H_x12_cross)
print('Kullback–Leibler divergence : Dkl(x1,x2) =\t',D_x12)
MI = np.zeros([nC,nC])
JE = np.zeros([nC,nC])
CE = np.zeros([nC,nC])
KL = np.zeros([nC,nC])
for i in range(nC):
x1 = X[:,i]
for j in range(nC):
x2 = X[:,j]
#Mutual Information
MI[i,j] = sp.mutual_Info(x1,x2)
#Joint entropy
JE[i,j]= sp.entropy_joint(x1,x2)
#Cross Entropy
CE[i,j]= sp.entropy_cross(x1,x2)
#Diff Entropy
KL[i,j]= sp.entropy_kld(x1,x2)
plt.figure(figsize=(10,10))
plt.subplot(221)
plt.imshow(MI,origin='lower')
plt.yticks(np.arange(nC),ch_names)
plt.xticks(np.arange(nC),ch_names,rotation=90)
plt.title('Mutual Information')
plt.subplot(222)
plt.imshow(JE,origin='lower')
plt.yticks(np.arange(nC),ch_names)
plt.xticks(np.arange(nC),ch_names,rotation=90)
plt.title('Joint Entropy')
plt.subplot(223)
plt.imshow(CE,origin='lower')
plt.yticks(np.arange(nC),ch_names)
plt.xticks(np.arange(nC),ch_names,rotation=90)
plt.title('Cross Entropy')
plt.subplot(224)
plt.imshow(KL,origin='lower')
plt.yticks(np.arange(nC),ch_names)
plt.xticks(np.arange(nC),ch_names,rotation=90)
plt.title('KL-Divergence')
plt.subplots_adjust(hspace=0.3)
plt.show()
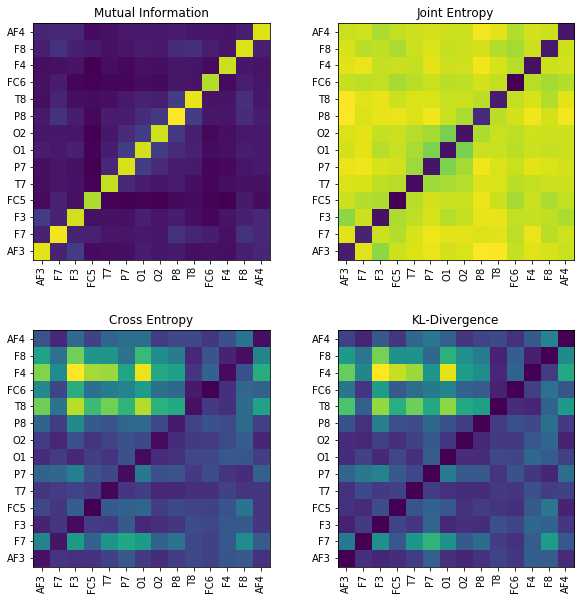